To have a great analytics culture, you need a great communications culture
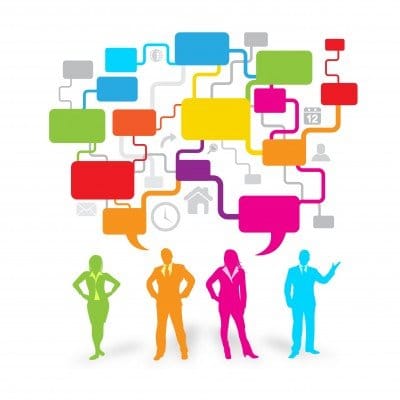
When you read about big data and/or data analytics projects and systems, it is rare that you also read bout communicating the outcome of those projects. Without the ability to communicate the results of any analysis to the broader business, most big data / analytics projects are doomed to mediocrity…or even failure.
The quantitative mind is a great one. It is one that I’m very familiar with and one that I wholeheartedly support. The ability to take a data set, analyze that data and create new information and knowledge from that data is an extremely important skill for people and organizations to have.
Just as important is the skill to be able to convert the outcome of any quantitative analysis into something that is easily digestible by people throughout an organization.
Take, for example, the world of academia. There are many really smart people performing research within universities and research facilities. These people conduct research and then publish the outcomes of that research in academic journals to share their new-found knowledge with others.
Have you ever picked up an academic journal/article? These articles are generally well-written and delivered in formal academic styles but they aren’t exactly ‘easy reading’. They are meant to be used for academic reporting within academic circles. They are also used within industry but most practitioners that read these journals and articles are usually people with similar education and experience as those folks who are writing / publishing these articles.
What happens when a finance manager picks up the Journal of Finance paper titled “Determinants of Corporate Borrowing?” Will they easily understand what the paper is trying to communicate? Let’s take a look at a portion of the abstract of the paper:
Many corporate assets, particularly growth opportunities, can be viewed as call options. The value of such ‘real options’ depends on discretionary future investment by the firm. Issuing risky debt reduces the present market value of a firm holding real options by inducing a suboptimal investment strategy or by forcing the firm and its creditors to bear the costs of avoiding the suboptimal strategy. The paper predicts that corporate borrowing is inversely related to the proportion of market value accounted for by real options. It also rationalizes other aspects of corporate borrowing behavior, for example the practice of matching maturities of assets and debt liabilities.
I would argue that anyone – given enough time – could understand what that paragraph is trying to communicate, but in the fast-paced world of business, does anyone really have time to sit down and study this paper? I doubt it. Most will call up a consultant and ask to help better understand the optimal approach to corporate debt. What is that consultant going to do? She will take her experience as a consultant (and in finance/banking), study the business, literature and best practices and then make a recommendation to the business on what they should do. If the consultant is any good, these recommendations will be provided in an easy to understand document that can be implemented effectively within the organization.
The same approach needs to be taken with data analytics. We can’t just throw a spreadsheet or chart over the wall at the business and expect them to understand what the data is telling them or what they should with that data. I see a lot of this these days though. A company will implement a new big data project, perform some analysis of the data and then provide the output of the analysis in pretty charts and tables but very rarely are there deep, meaningful discussions and analysis about what that data is really telling the business and/or what the business should do based on the data analysis.
Now, you may say that good data scientists / analysts already do this…and you’d be right. But, not everyone is a great analyst nor is it a skill set that most organization’s are hiring for these days. When I talk to clients about big data, they talk about the need to get the best hardware, software and analytical skills…but they rarely talk about the need to find great communicators.
Companies regularly spend millions of dollars on the ‘hard’ costs for big data and data analytics. They’ve even begun spending a good deal of money on the ‘soft’ costs to get their people the best training available so they can be the best data analysts available but it is rare that they spend much money on communications training.
The funny thing about this particular topic is that most data scientists consider themselves to be good communicators. In my experience, the really good ones are…but the majority of the ‘new’ data scientists struggle with this aspect of their job.
If you want to be a great data scientist, become a great communicator and storyteller. As a data scientist, if you can’t communicate in a way that is informative and useful to the business, the work you do in the ‘quant’ world isn’t that valuable to the company. The same can be said to the business in general – if you want a great data analytics culture, build a great communications culture. You can’t have one without the other.
Comments ()